Sentiment analysis is a fascinating field that explores the complex task of understanding emotions and opinions in text. In this article, we will dive into the world of sentiment analysis, its importance in natural language understanding, and its applications in various industries. We will also discuss the challenges that arise when analyzing sentiment and the ongoing efforts to improve sentiment analysis tools.
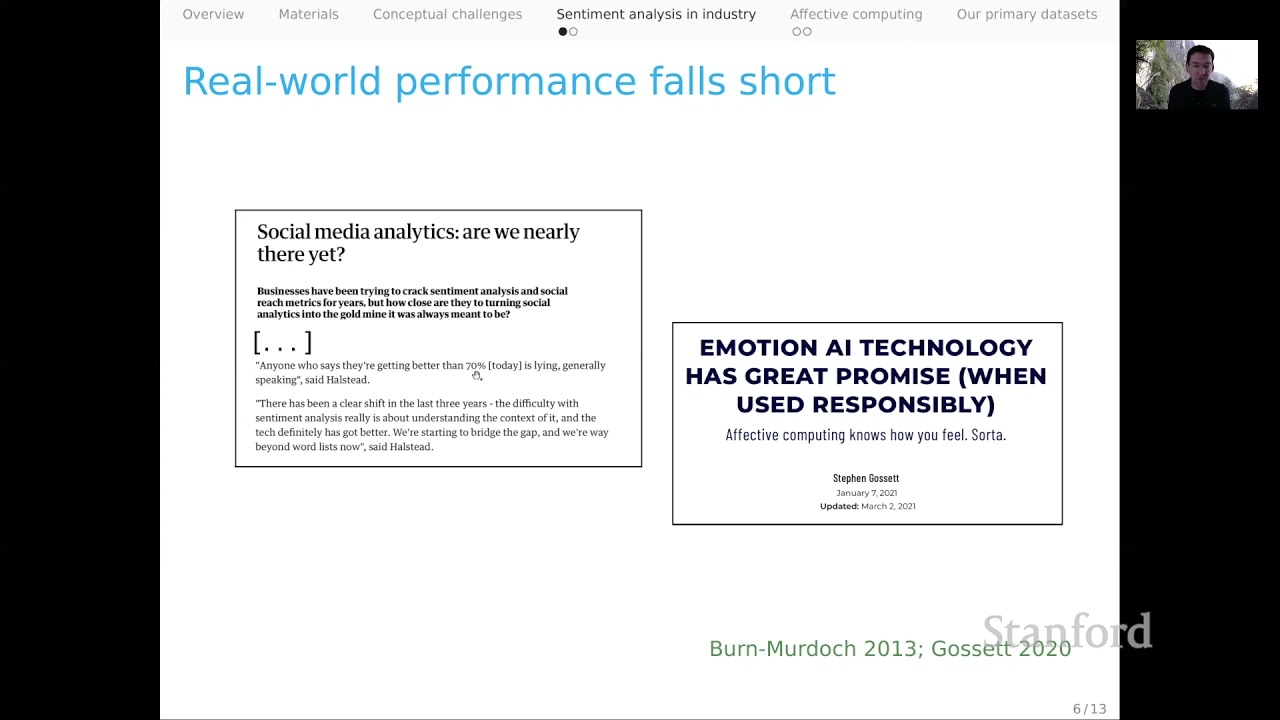
Contents
Understanding Sentiment Analysis
Sentiment analysis is the process of determining the sentiment expressed in a piece of text, whether it is positive, negative, neutral, or even something else. At first glance, it might seem easy to identify sentiment in a sentence, but it quickly becomes apparent that it is a much deeper and more complex problem.
Consider the sentence, “There was an earthquake in California.” Although many sentiment analysis systems might assign a negative sentiment to this sentence, it is important to question whether it truly expresses sentiment or merely states a fact. This ambiguity highlights the challenges faced by sentiment analysts in accurately determining sentiment in text.
The Challenge of Subjectivity
Sentiment analysis becomes even more challenging when subjective evaluative perspectives are involved. For example, let’s analyze the sentence, “The team failed to complete the challenge.” Is this sentence positive or negative? While it does imply more than just a statement of fact, the sentiment depends on the perspective of the speaker. Is the speaker advocating for the team or against it? Deciphering the underlying sentiment requires considering the context and the speaker’s viewpoint.
The Complexity of Language
Language can be a tricky landscape for sentiment analysis. Sentiment expression often goes beyond literal use of language and incorporates subjective and emotional elements. For instance, consider the sentence, “The party fat cats are sipping their expensive imported wines.” Although it contains some positive language, the overall sentiment might be negative. Sentiment analysis systems need to navigate these nuances to accurately capture the sentiment conveyed.
Sentiment Analysis in Industry
Sentiment analysis has gained significant traction in industry, especially in areas like marketing and customer experience. The ability to gauge customer sentiment and opinions has become vital for businesses. However, despite advancements in data-driven approaches, sentiment analysis tools still face limitations.
Business leaders often desire sentiment analysis tools that provide simple visualizations, such as pie charts with percentages of positive, negative, and neutral sentiments. However, merely classifying texts into these categories and presenting change over time is insufficient for decision-making. To provide meaningful insights, sentiment analysis tools need to delve deeper into the underlying factors and understand the customers’ sentiments beyond the gross classifications.
The Future of Sentiment Analysis
Expanding the scope of sentiment analysis beyond binary classifications into a more nuanced understanding of emotions is an exciting area of research. Affective computing encompasses sentiment analysis and considers other emotional aspects in language. This multidimensional space presents opportunities to explore related tasks like bias detection, stance taking, hate speech identification, and more.
In this unit, we will primarily focus on sentiment analysis using two popular datasets: the Stanford Sentiment Treebank (SST) and DynaSent. These datasets provide diverse perspectives, analyzing sentiments in movie reviews and product/service reviews, respectively. By combining these datasets, we aim to gain insights into sentiment analysis and develop systems that can transfer knowledge across domains effectively.
FAQs
Q: What is sentiment analysis?
A: Sentiment analysis is the process of determining the sentiment expressed in a piece of text, whether it is positive, negative, neutral, or something else.
Q: Why is sentiment analysis important?
A: Sentiment analysis has significant implications in various industries, such as marketing and customer experience. It helps businesses understand customer sentiments and make informed decisions based on the analysis.
Q: What are the challenges in sentiment analysis?
A: Sentiment analysis faces challenges due to the subjective nature of sentiment expression, the complex use of language, and the need to capture nuanced emotions accurately.
Q: Are sentiment analysis tools effective?
A: While sentiment analysis tools have made advancements, there is still room for improvement. The tools should aim to provide deeper insights beyond simple categorizations of sentiment.
Conclusion
Sentiment analysis is a complex and intriguing field that explores the depths of understanding emotions in language. By delving into the challenges of sentiment analysis and the ongoing efforts to enhance sentiment analysis tools, we can gain a deeper understanding of sentiments expressed in text. As sentiment analysis continues to evolve, it holds the potential to revolutionize various industries by providing valuable insights into customer opinions and emotions.
For more information about sentiment analysis and other exciting areas of technology, visit Techal.