Welcome back! In this article, we will delve into some fascinating case studies in the aerospace sector that showcase the power of data-intensive methods, machine learning, and visualization. These case studies, done through collaborations between the University of Washington and Boeing, provide a glimpse into the vast potential of these technologies in the aerospace industry.
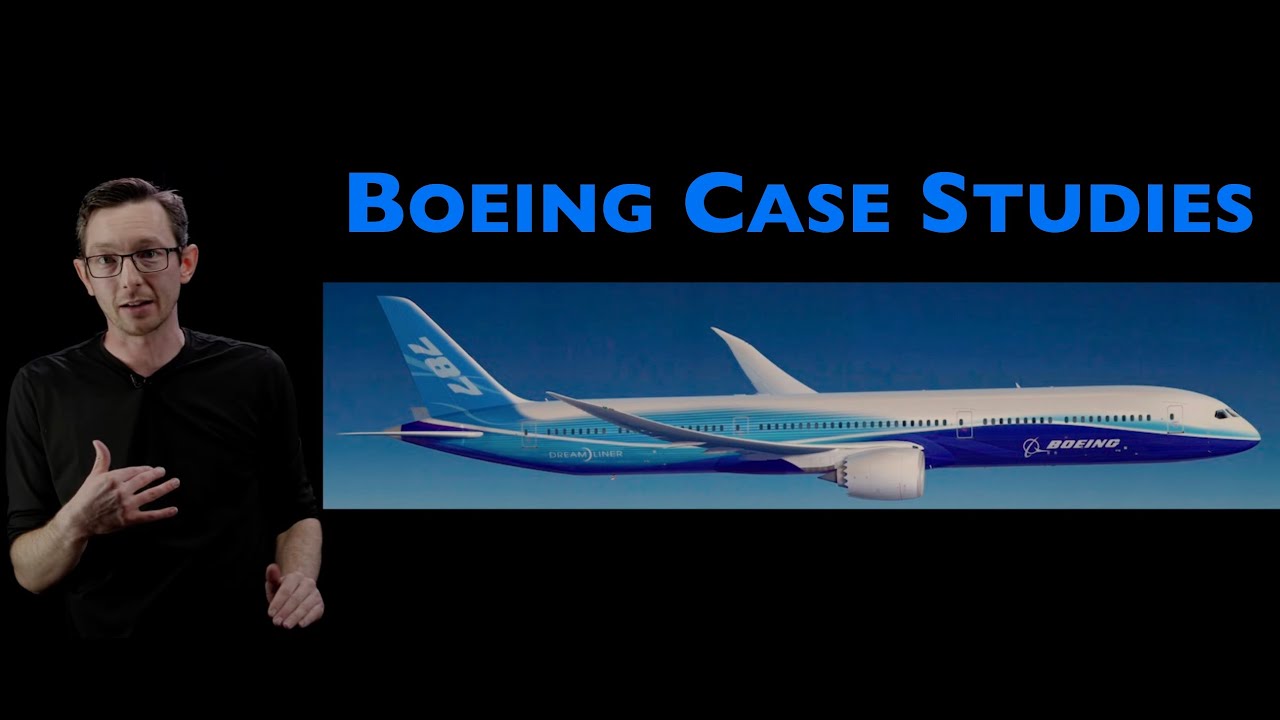
Contents
Reducing the Number of Unique Parts on an Aircraft
One of the eye-opening examples is the effort to reduce the number of unique parts, specifically brackets, on an aircraft. Modern aircraft like the Boeing 787 consist of tens of thousands of unique brackets, each designed for different purposes such as holding cables or bracing. Instead of scouring through a catalog of thousands of brackets, engineers often find it easier to design new brackets for specific cases, leading to a proliferation of brackets.
To address this issue, a collaboration between the University of Washington and Boeing engineers sought to identify a core subset of brackets that could perform well across multiple use cases. By leveraging machine learning and data science techniques, they took a database of brackets used in commercial aircraft and used clustering algorithms to find brackets that were similar to many others. This allowed them to consolidate and standardize brackets, resulting in a significant reduction in the number of unique parts. This not only saves engineers time but also reduces costs and simplifies inventory management.
Image Source: Techal
Streamlining Flight Testing Through Data Intensive Solutions
Flight testing is a crucial and extensive process in certifying aircraft. To streamline and improve this process, data-intensive solutions have been employed, leveraging the power of extensive sensor data collection and analysis. Aircraft undergoing flight tests are equipped with thousands of sensors, measuring various parameters to ensure rigorous testing and certification.
One example of streamlining flight testing is drag estimation. Traditionally, aircraft were tested in optimal weather conditions to obtain accurate drag profiles. However, waiting for perfect weather conditions can result in significant delays and increased costs. Through collaboration between the University of Washington and Boeing, historical flight test data was utilized to develop machine learning models that could compensate for imperfect weather conditions. By leveraging the patterns and correlations present in the data, these models could estimate drag as accurately as if the tests were conducted under ideal weather conditions.
Another example is anomaly detection. During flight tests, sensors occasionally fail or produce unreliable data. This can lead to wasted time and resources if not identified promptly. However, due to the redundancy and multitude of sensors installed on the aircraft, it is possible to detect anomalies in real-time. By utilizing statistical patterns and correlations among different measurements, anomalies can be flagged, enabling prompt corrective actions and, in some cases, even filling in missing or faulty data.
Image Source: Techal
The Power of Digital Twin
In the aerospace industry, companies like Boeing possess vast amounts of data accumulated throughout the entire lifecycle of their products. This wealth of data, ranging from initial concepts to service records, can be leveraged to create digital twins. A digital twin is a model that encapsulates the entire asset, such as an aircraft or a factory, pinned to the actual data collected over its lifetime.
The digital twin approach allows for model-based engineering and optimization, enabling the design of safer, higher-performing aircraft in a more efficient manner. With the integration of reduced order modeling, uncertainty quantification, machine learning, sensor fusion, and the internet of things, this approach revolutionizes the design and optimization processes, reducing the need for extensive physical testing and resulting in faster and more resource-efficient outcomes.
Image Source: Techal
FAQs
Q: Are these case studies exclusive to Boeing aircraft?
A: While these case studies involve collaborations with Boeing, the concepts and methodologies can be applied to the aerospace industry as a whole.
Q: How do these technologies benefit customers?
A: By streamlining processes, reducing costs, and optimizing designs, these technologies ultimately result in safer and more efficient aircraft, thus benefiting customers.
Q: Are there other areas where these technologies can be applied?
A: Absolutely! These technologies have wide-ranging applications beyond just bracketing and flight testing. They can be used in designing electrical connectors, manufacturing processes, and even in the development of new materials and aerodynamic innovations.
Conclusion
The collaboration between the University of Washington and Boeing showcases the immense possibilities that arise when data-intensive methods, machine learning, and visualization are applied in the aerospace industry. From reducing unique parts to streamlining flight tests and harnessing the power of digital twins, these technologies pave the way for safer, higher-performing aircraft, all while minimizing costs and optimizing resource allocation.
To learn more about the exciting developments in the world of technology, visit Techal.
Original source: Techal