Welcome to Techal’s comprehensive guide on using linear models for t-tests and ANOVA! In this article, we will explore how to apply the concepts of linear regression to perform t-tests and analyze variances.
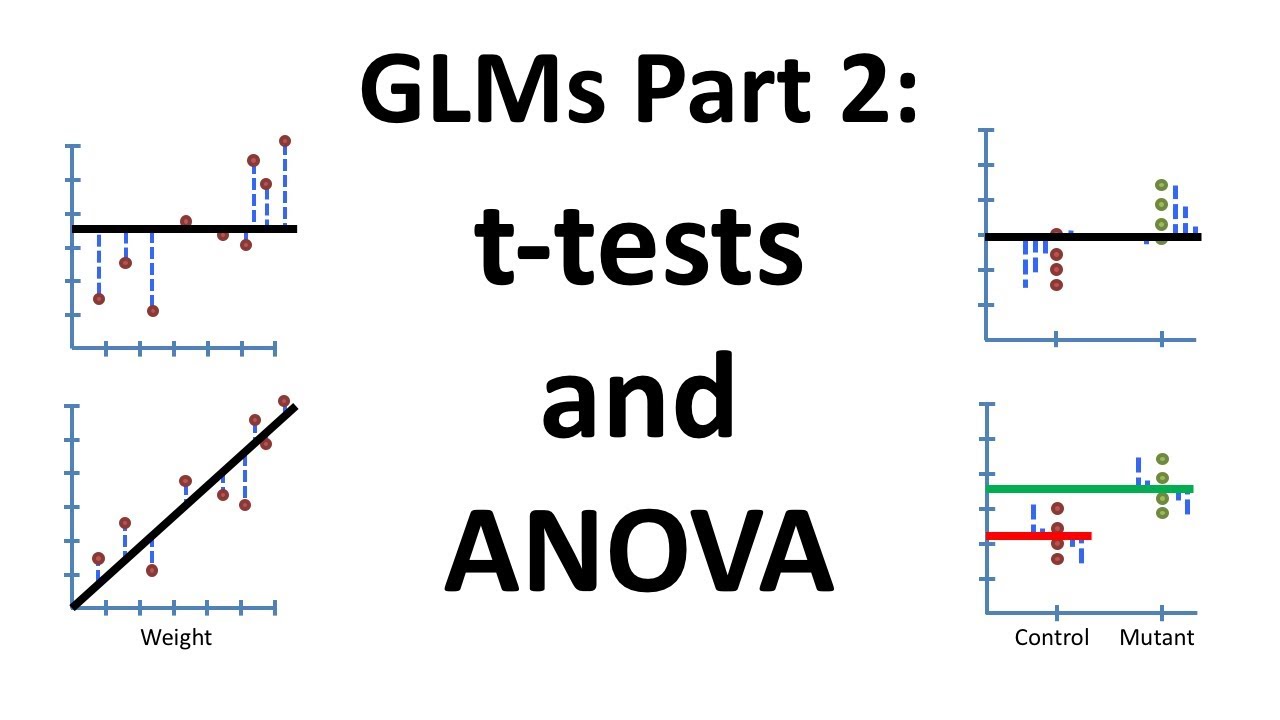
Introduction
Linear models are versatile tools that can be used for various statistical analyses. Previously, we discussed how linear regression can help us predict outcomes based on independent variables. Now, we will dive deeper into how linear models can enable us to compare means and determine if they are significantly different from one another. We will walk you through the steps, using the example of gene expression analysis in control and mutant mice.
Comparing Means: T-tests
T-tests are commonly used to compare means between two groups. In our example, we are comparing the gene expression levels in control mice and mutant mice. The goal is to determine if these means are significantly different from each other.
To perform a t-test using linear models, we can leverage the same techniques we employed in linear regression. Let’s break down the steps.
Step 1: Find the Overall Mean
To focus solely on the outcome variable (gene expression) on the y-axis, we ignore the x-axis. By calculating the overall means for both groups, we can proceed with the analysis.
Step 2: Calculate the Sum of Squared Residuals Around the Mean
Next, we calculate the sum of squared residuals around the mean. These residuals represent the distances between the data points and the overall mean. This step helps us understand the variability within each group.
Step 3: Fit a Line to the Data
Now, we shift our focus back to the x-axis and fit lines to the data. For the control group, the least squares fit is simply the mean itself. Similarly, for the mutant group, the mean represents the best fit line. By combining these lines into a single equation, we create a flexible way to handle more complex situations.
Step 4: Calculate the Sum of Squares of the Residuals Around the Fitted Lines
We calculate the sum of squares of the residuals around the fitted lines. This step allows us to quantify the remaining variability in the data and assess the goodness of fit for our model.
Step 5: Calculate F and the p-value
With the sums of squares in hand, we can now calculate F and derive a p-value. The p-value indicates the likelihood of observing the differences between the means due to chance alone. If the p-value is below a certain threshold (typically 0.05), we can conclude that the means are significantly different.
Analyzing Variances: ANOVA
ANOVA (Analysis of Variance) is a statistical technique used to compare means across multiple groups. In our example, we will expand our analysis to include control mice, mutant mice, mice on a specialized diet, and heterozygote mice.
Step 1: Calculate the Sum of Squares Around the Mean
Just like in the t-test scenario, we start by calculating the sum of squares around the mean. By obtaining an overall mean value for all categories and summing up the squared residuals, we gain insights into the total variability in the data.
Step 2: Calculate the Sum of Squares Around the Fitted Lines
Next, we calculate the sum of squares around the fitted lines. In this case, we have different means for each category, resulting in multiple lines. By estimating the fit for each line, we can assess the variation within each group.
Step 3: Determine the Parameters and Create a Design Matrix
To simplify the calculations, we determine the number of parameters in the equation for each mean and create a design matrix. This matrix acts as a switchboard, turning the means on and off for different categories. With the design matrix in place, we can represent the fit to the data using a single equation.
Step 4: Calculate F and Derive the p-value
Now, armed with the sums of squares around the mean and the fitted lines, as well as the number of parameters, we can calculate F and derive a p-value. The p-value indicates the likelihood of observing the differences in means across the categories due to chance alone. If the p-value is below the established threshold, we can conclude that there is a significant difference between the means.
FAQs
Here are some frequently asked questions about using linear models for t-tests and ANOVA:
-
What is the purpose of linear models in statistical analysis?
Linear models offer a powerful framework for predicting outcomes, comparing means, and analyzing variances in a wide range of statistical analyses. -
Can linear models be applied to more complex scenarios than t-tests and ANOVA?
Absolutely! Linear models are adaptable and can handle various scenarios, including multiple independent variables, interactions, and non-linear relationships.
Conclusion
In this comprehensive guide, we explored how linear models can be used for t-tests and ANOVA. By leveraging the concepts of linear regression, we can gain deeper insights into the differences between means and variances. Understanding these statistical techniques empowers us to make informed decisions and draw meaningful conclusions from our data.
Stay tuned for more exciting guides and analyses from Techal! If you enjoyed this article, please consider subscribing to our newsletter for future updates and feel free to leave any suggestions or questions in the comments below.
Techal – Empowering You with Technological Knowledge