Welcome to a mind-boggling journey through the world of virus models and the dangers of p-hacking! In this thrilling live stream, I, Josh Dharma, your trusty host, will unravel the mysteries and reveal the hidden truths. So buckle up and prepare to be amazed!
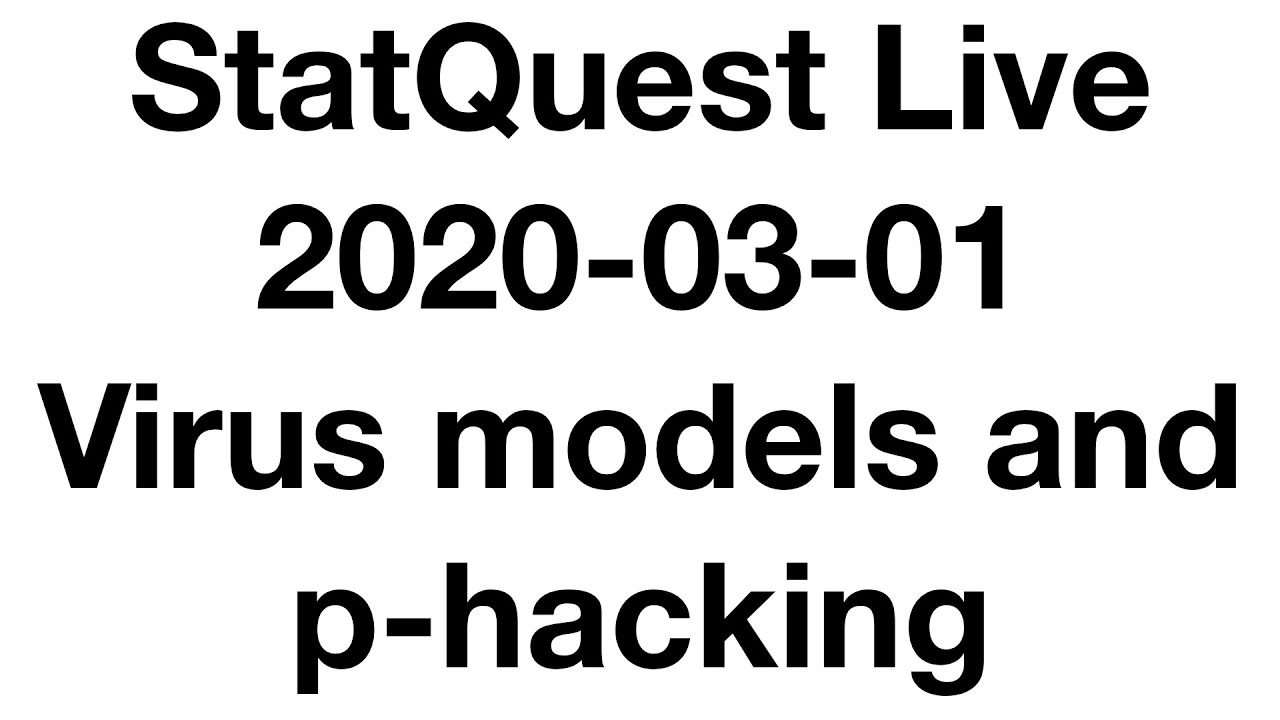
Contents
The Power of Virus Models
Imagine predicting the growth of a new coronavirus epidemic. Sounds like a daunting task, right? Well, it is! The best models for this predicament are based on differential equations. These models take into account various factors, such as social networks, disease transmission characteristics, and contagion rates. But wait, differential equations? Don’t be intimidated! They are actually quite fun and intuitive, thanks to computers doing most of the work (I mean, who doesn’t love that?). Although I won’t be diving into differential equations in detail in this session, I promise, we’ll tackle some exciting topics like neural networks and time series. But hey, if there’s a massive demand for differential equations, who knows? Maybe we’ll give it a shot!
The Perils of P-Hacking
Now, let’s explore the treacherous world of p-hacking. Picture this: you’ve conducted an experiment with four groups of subjects, each on a different diet. After measuring their weight gain over four weeks, you notice that Diet C stands out. It’s tempting to jump the gun and conclude that Diet C made a significant difference compared to the others. But hold on! That would be a grave mistake called p-hacking.
Why is it a mistake? Because p-hacking involves cherry-picking data that looks different and testing only those groups. This can lead to false positives, where we are tricked into believing some groups are significantly different when they are not.
To demonstrate this, let’s take a hypothetical example. We measure the recovery time of individuals from an illness and plot it on a graph. Two groups of three people each show similar recovery times within 5 to 15 days (95% probability). If we compare their means statistically, we obtain a p-value of 0.86, indicating no significant difference.
Now, let’s randomly pick another set of three people from the same distribution and compare their recovery times to the previous group. Again, we find no significant difference (p-value of 0.63). If we keep repeating this process, sooner or later, we might stumble upon a pair with a p-value lower than 0.05, indicating a false positive.
P-hacking also extends to trying multiple tests after data collection. It’s tempting to increase the sample size or perform additional experiments until we get a significant result. However, this behavior leads to an increased risk of false positives.
The Power of Power Analysis
To avoid the pitfalls of p-hacking, we need a plan! Enter power analysis. Picture two distributions with little overlap. By collecting data from both distributions, we plot the means on a graph and obtain a p-value below 0.05. This represents a true positive, where we correctly reject the hypothesis that the samples come from the same distribution. This is what we want!
Power analysis helps us determine the sample size needed to ensure a high probability (80% in most cases) of correctly rejecting the null hypothesis. By conducting such an analysis beforehand, we can avoid the temptation to add more data after observing borderline p-values.
So, next time you encounter a p-value slightly above 0.05, resist the urge to collect more data and cross your fingers. Instead, perform a power analysis using your existing data to determine the appropriate sample size for a high probability of success.
Wrapping Up and Future Adventures
Congratulations, my friends! You have now journeyed through the mesmerizing realms of virus models and p-hacking. But fear not, for this is only the beginning! Our future adventures together hold the promise of exploring Bayesian statistics, fixed effects versus random effects, and countless other captivating topics. So stay curious, keep questing, and together we shall conquer the fascinating world of information technology!
Until next time, my fellow adventurers. Keep questing and never stop seeking knowledge.
This article is brought to you by Techal – your ultimate guide to all things tech!