Welcome back to another fascinating journey into the realm of technology! In this article, we will delve into the captivating world of the Singular Value Decomposition (SVD) and Eigenfaces. You are in for a treat as we explore the extraordinary power of these concepts through an intriguing example involving two iconic action heroes. Get ready to witness the magic unfold before your very eyes!
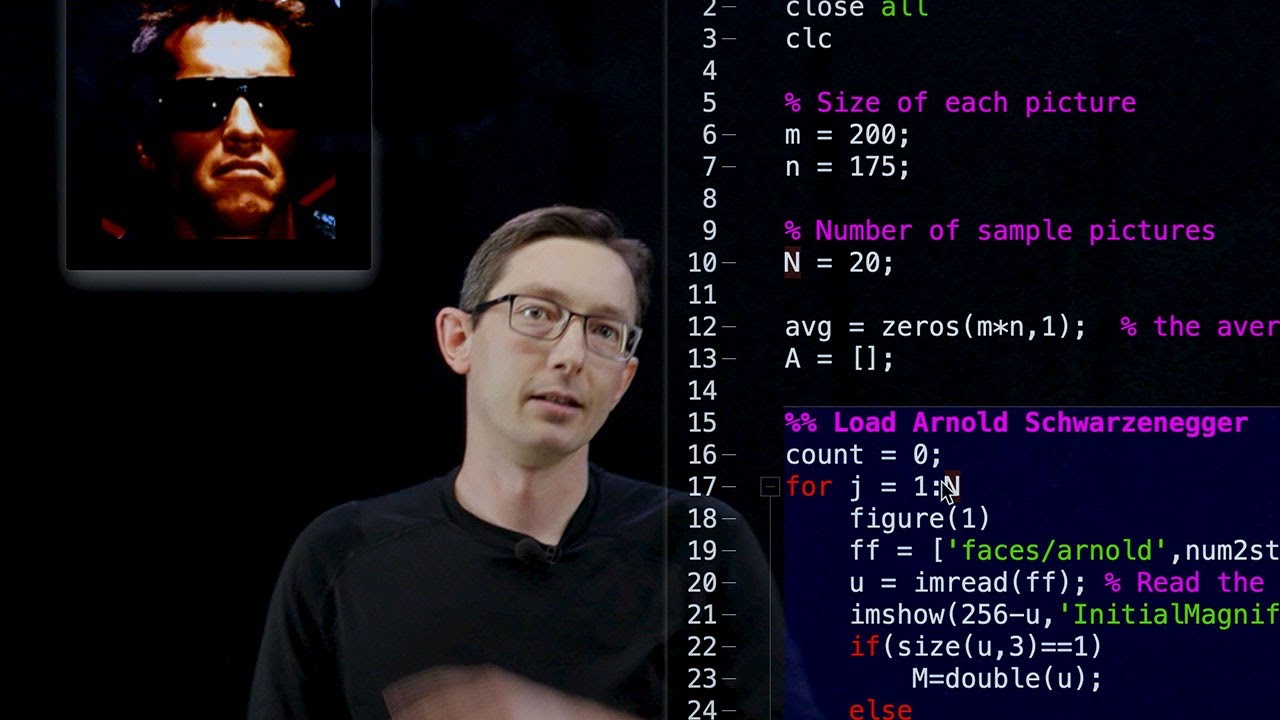
Contents
- Unveiling the Eigen Heroes
- Discovering the Eigenfaces
- Unveiling the Average Action-Hero
- The Power of Subtraction and Principal Component Analysis
- Behold, the Eigen Action Heroes
- Projecting Images into Eigenface Space
- Clustering and Classification
- The Enigmatic Realm of Classification
- Unraveling the Unexpected
- Reflecting on the Shallow Nature of Image Classification
- The Quest for Enhanced Accuracy
- Embracing the Power of Eigen Action Heroes
- FAQs
- Conclusion
Unveiling the Eigen Heroes
In this example, we will embark on a quest to discover the Eigenfaces of two legendary actors: Arnold Schwarzenegger and Sylvester Stallone. This enthralling experiment will enable us to cluster these larger-than-life personalities within the vast realm of Eigenface space. So, let’s dive right in and explore the wonder of this fascinating process.
Discovering the Eigenfaces
The code for this example is relatively simple and closely resembles that of other Eigenface implementations. First, we gather a collection of images featuring the action heroes. These images undergo cropping and alignment to ensure consistency in facial positioning. Each image is a 200 by 175 grayscale image. We then assemble these images into a matrix, with the first 20 columns representing Arnold and the subsequent 20 columns representing Stallone.
Now, let’s load the images and convert them to grayscale if necessary. We will plot them on the screen, pausing briefly to appreciate their magnificence. As we progress through the images, we will calculate the average face by adding up all the faces and dividing by the total count. This process allows us to build a comprehensive average representation.
Unveiling the Average Action-Hero
Behold, the moment has arrived! We are about to witness the creation of the average action-hero face. Brace yourself as we plot this remarkable result for your eyes to behold. The average action-hero face is a fascinating blend, encapsulating the heroic essence of the individuals we admire.
The Power of Subtraction and Principal Component Analysis
Prepare to be amazed! We are about to embark on a journey that involves subtracting the average face from each individual image. This subtraction process facilitates principal component analysis, enabling us to identify the vital components that define an action hero.
Through this enthralling process, the magic begins to unfold. We plot the singular values (denoted as s
) and compute the Singular Value Decomposition (SVD) of the resulting matrix. Each column of the u
matrix represents our esteemed eigen action heroes. These extraordinary entities come to life, showcasing their unique characteristics.
Behold, the Eigen Action Heroes
As we gaze upon these incredible eigenfaces, we are captivated by their essence. Each face is a linear combination of the images we fed into the system. The first few dominant eigen action heroes lay the foundation, while the subsequent ones reveal intricate features. We can observe fascinating attributes, including Terminator glasses, Stallone’s piercing eyes, and the average face that emerges as a familiar presence.
Projecting Images into Eigenface Space
Prepare to witness the true power of Eigenfaces! We can take any image from our library and project it into the realm of Eigenface coordinates. As we project each image into the principal components, we transform a high-dimensional vector into a three-dimensional representation based on the dominant eigenfaces. These coordinates provide insights into the image’s composition and its relationship to the eigenfaces.
Clustering and Classification
The real excitement begins now! A magical process awaits us—one that involves clustering and classification. By assigning color-coded markers to each image’s coordinates, we can visually differentiate between Arnold and Stallone.
Upon observing the plotted data, a pattern emerges. The blue dots represent Arnold, while the yellow dots represent Stallone. A distinct separation becomes apparent, validating the uniqueness of these iconic action heroes. However, it is essential to note that there is some overlap in the distributions, indicating a subtle similarity between them.
The Enigmatic Realm of Classification
Now, let’s explore the captivating world of image classification. Imagine having a test image that was not part of the training data. We can project this image into the eigenface coordinates and observe which cluster it aligns with—Arnold or Stallone. This basic idea forms the foundation of image classification.
To illustrate this concept, we present two intriguing test images—a hybrid of Harry Potter and Stallone, and an image of the TV Terminator, Summer Glau, who may bear a resemblance to Arnold. When we project these test images into eigenface space, we discover that they align remarkably well with their respective clusters. The TV Terminator image falls within Arnold’s cluster, while the Harry Potter Stallone hybrid aligns perfectly with the Stallone cluster.
Unraveling the Unexpected
Prepare to be mystified by the unexpected! We are about to embark on a thrilling experiment involving Taylor Swift and the enigmatic world of image classification. Through the same process, we load a collection of Taylor Swift images and project them alongside Stallone’s.
As the results unfold, you may find yourself astounded. The separation between Taylor Swift and Stallone exceeds that of Arnold and Stallone. It appears that Taylor Swift’s distinctive features project further away from the Stallone cluster, indicating a higher dissimilarity. This surprising outcome challenges common perceptions and exposes the complexity of image classification.
Reflecting on the Shallow Nature of Image Classification
As we delve deeper into the intricacies of image classification, we discover certain limitations within these classification techniques. Skin tone and hair color play a significant role in determining the correlation between faces. In the case of Taylor Swift and Arnold Schwarzenegger, their fair skin and blonde hair contribute to a higher correlation, despite their gender differences. This realization prompts us to reflect on the shallowness of these naive image classification approaches.
The Quest for Enhanced Accuracy
In the pursuit of enhanced accuracy, technology continues to evolve. Companies like Facebook have employed more advanced algorithms that incorporate three-dimensional information gathered from two-dimensional images. By inferring the three-dimensional geometry of facial features, a higher level of accuracy can be achieved.
For instance, Facebook utilized shadows and depth information to map two-dimensional images onto a three-dimensional model, mimicking the human capacity for understanding the three-dimensional world. This breakthrough led to a significant improvement in their facial recognition algorithms, paving the way for more accurate image classification.
Embracing the Power of Eigen Action Heroes
In conclusion, the world of Eigenfaces and the Singular Value Decomposition holds incredible power. The ability to cluster and classify images based on their eigenface coordinates opens up a realm of possibilities. Despite the limitations and complexities, this captivating field continues to spark wonder and curiosity among technology enthusiasts.
We invite you to explore this mesmerizing world further and unleash your creativity. Feel free to experiment with your own images and witness the magic of Eigen Action Heroes. For more thrilling insights into the fascinating realm of technology, visit the Techal website at Techal.
FAQs
-
What is the Singular Value Decomposition (SVD)?
The Singular Value Decomposition (SVD) is a matrix factorization method that breaks down a matrix into three matrices: U, Σ, and V. It is widely used in various fields, including image processing and data analysis. -
What are Eigenfaces?
Eigenfaces are the eigenvectors of the covariance matrix of a set of face images. They represent the most significant features that define a face, allowing for face recognition and classification. -
How does image classification work using Eigefaces?
Image classification using Eigenfaces involves projecting an image onto the eigenface coordinates and determining which cluster it aligns with. This classification technique relies on the similarity between the projected image and the clusters of known images.
Conclusion
We hope this journey into the world of Eigen Action Heroes has ignited your passion for technology and kindled your curiosity about the intricacies of image classification. The power of Eigenfaces and the Singular Value Decomposition is truly remarkable, offering a glimpse into the potential that lies within the digital realm. As technology continues to advance, we eagerly await the next thrilling breakthrough that will shape the future of image processing and analysis. Until then, keep exploring and embracing the wonders of technology!