Welcome back, tech enthusiasts! Today, we dive into the fascinating world of predictive shimming at Boeing. Shimming, the art of using filler components to bridge gaps between parts during assembly, has long been a labor-intensive and expensive process. However, Boeing has revolutionized this technique by leveraging predictive shimming, ushering in a new era of efficiency and cost savings.
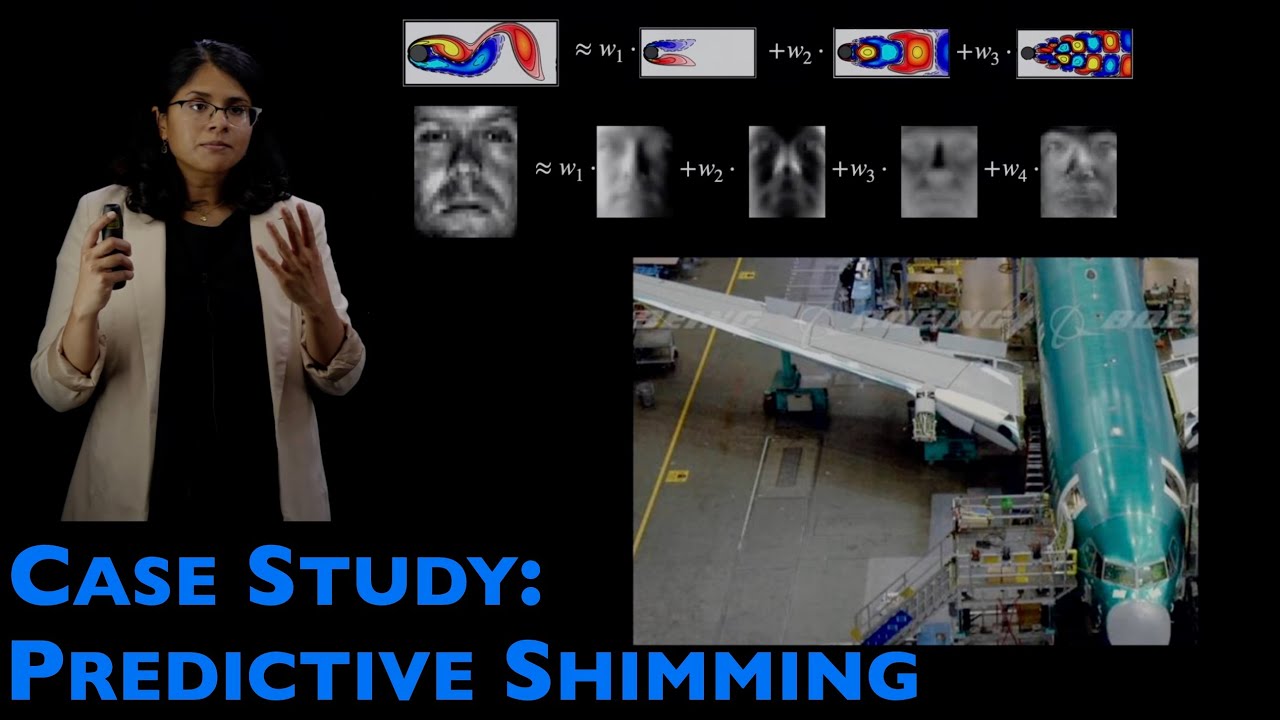
Contents
What is Predictive Shimming?
Traditionally, shimming has been a reactive process where complex parts are dry-fitted together, gaps are measured by hand, shims are manufactured, and parts are reassembled. However, for intricate structures like winged body joints, this method is computationally expensive and time-consuming.
Predictive shimming, on the other hand, involves scanning the interior surface of one part and the exterior surface of another. By digitally aligning these surfaces using computational methods, gaps can be measured and accurately predicted in a virtual environment. This data is then used to manufacture shims precisely tailored to the specified gaps, saving both time and resources.
The Power of Historical Data
Can historical data from previous aircraft be used to streamline the predictive shimming process? That’s the question Boeing aimed to answer for the 787-8 and dash 9 winged body join. The team compiled an extensive dataset of 10,000 gap measurements from the metrology procedure for 50 different aircraft.
The goal was to reduce measurement requirements and achieve substantial cost savings during manufacturing. The shimming process is critical, and any time or resource saved directly translates to millions of dollars saved per aircraft.
Learning from Data: Principal Component Analysis
Boeing employed a machine learning technique called principal component analysis (PCA) to find patterns within the vast dataset of shim gaps. This is similar to image processing or facial recognition, where high-dimensional data, such as a person’s face, can be reduced to a set of features or eigenfaces.
By extracting dominant features from the data, Boeing was able to determine targeted gap measurements that would allow for the accurate prediction of shim gaps. These sparse sensor measurements became the foundation for solving a system of equations to recover the full image or, in this case, the shim gaps of a new aircraft.
Robust PCA for Enhanced Precision
To enhance the accuracy of their predictions, Boeing implemented robust PCA. This technique isolates outliers within the data that may not represent the overall gap distribution. By filtering out these outliers, the remaining gap measurements reflect a more structured distribution, aligning with expected manufacturing tolerances.
Achieving Precision and Efficiency
Through extensive optimization and analysis, Boeing discovered that 99% of unmeasured gaps could be predicted with an accuracy within five thousandths of an inch, meeting manufacturing tolerances specified by the application. And all of this was accomplished with 30 times fewer measurements.
By strategically placing a targeted number of sensors along different segments of the winged body joint, Boeing successfully reconstructed nearly a thousand unmeasured gaps using only 20 to 25 shim locations. The fidelity of these predictions was exceptionally high, almost reaching 98% to 99%.
Validation: Optimized Sensors vs. Random Point Sensors
To validate the effectiveness of their optimized sensor approach, Boeing compared it to a data-agnostic approach using random point sensors. The results were clear: the optimized sensor approach outperformed random measurements.
The probability distribution of gap prediction errors showed that with optimized measurements, the majority of the distribution’s mass was within the manufacturing tolerance of five thousandths of an inch. In contrast, using random measurements, nearly half of the probability mass exceeded this tolerance.
FAQs
Q: Can predictive shimming be applied to other aircraft or structures?
A: Yes, the principles and techniques utilized in predictive shimming can be adapted and applied to various aircraft and structural components.
Q: Are there any limitations to predictive shimming?
A: While predictive shimming offers significant advantages, it’s important to ensure that historical data is representative and that the selected sensors capture the necessary gaps accurately.
Q: How does predictive shimming impact manufacturing costs?
A: By reducing measurement requirements and streamlining the shimming process, predictive shimming can result in substantial cost savings during aircraft manufacturing.
Conclusion
Boeing’s groundbreaking work in predictive shimming has transformed the aerospace industry, driving efficiency, and cost savings. By leveraging historical data, applying machine learning techniques like PCA, and optimizing sensor placement, gaps in complex structures can be accurately predicted with fewer measurements and in a fraction of the time.
This innovation not only enhances manufacturing precision but also paves the way for advancements in other areas of aerospace engineering. Predictive shimming empowers engineers with a powerful tool to optimize production processes and achieve unparalleled efficiency in aircraft assembly.
Thank you for joining us today on this enlightening journey into predictive shimming. To stay updated on the latest technological breakthroughs, visit Techal. Stay curious, stay tech-savvy!