Imagine having the power to classify images using a simple and efficient approach. Well, in this article, we will explore the fascinating concept of eigenfaces and how they can be used for image classification. Buckle up as we take you on an exhilarating journey into the world of dimensionality reduction and facial recognition.
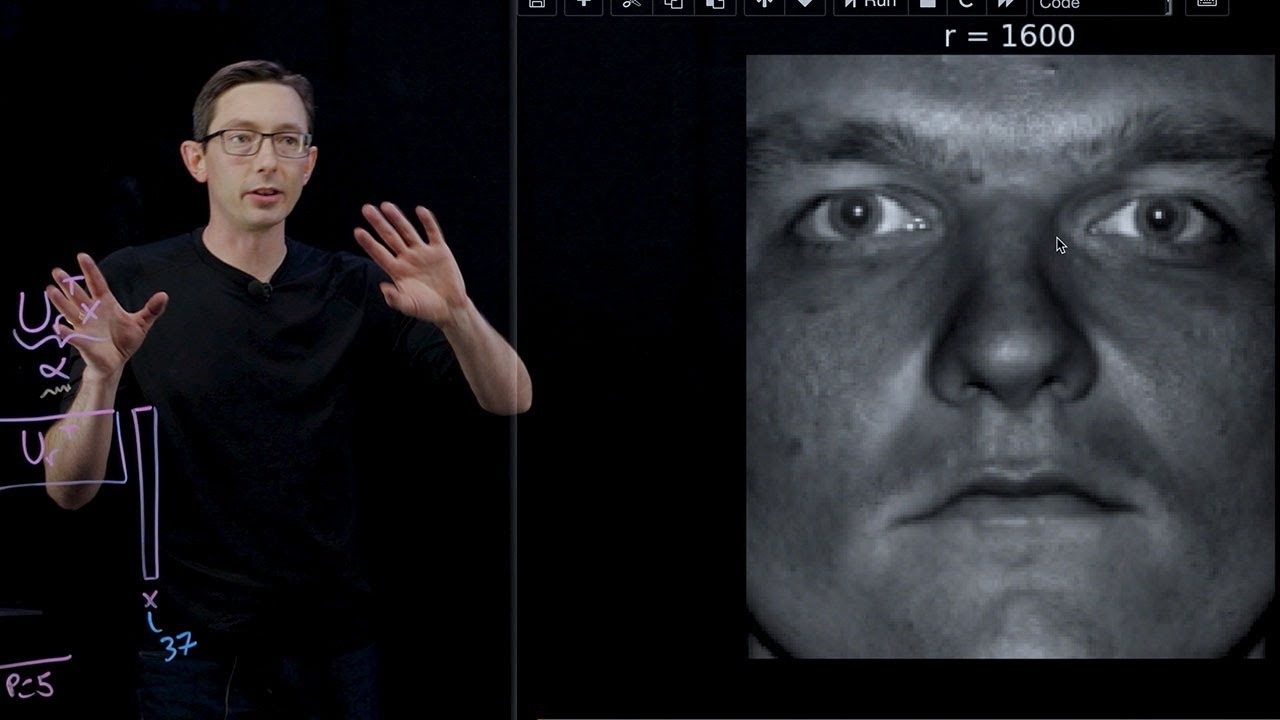
Contents
The Essence of Eigenfaces
Eigenfaces are a product of the singular value decomposition (SVD), a mathematical technique that decomposes a matrix into its constituent parts. In the case of eigenfaces, we reshape a library of people’s faces into column vectors and perform the SVD. The result is a set of orthonormal eigenfaces, forming a basis to represent human faces.
Image Classification Made Easy
So, how can eigenfaces aid in image classification? Instead of performing classification in a high-dimensional megapixel image space, we can project an image onto the eigenface basis. By projecting an image onto the first R-dimensional eigenface coordinates, we obtain a compact representation known as the alpha vector. This vector serves as a unique “fingerprint” for a person’s face in this low-dimensional space.
Clustering for Classification
The power of eigenfaces lies in their ability to enable clustering and subsequent classification. By randomly selecting individuals from our face library and projecting their faces onto specific eigenface dimensions, we can observe how well they cluster in this reduced subspace.
For instance, let’s consider person two and person seven. By projecting their faces onto the fifth and sixth principal components, we can visualize their clustering in a two-dimensional space. Person two’s images tend to cluster together in one region, while person seven’s images form another distinct cluster. This clustering enables us to build an accurate and efficient classifier.
The Beauty of Principal Components
It’s worth noting that the choice of principal components directly impacts the classification accuracy. In our example, we deliberately selected the fifth and sixth principal components instead of the first two. The first few principal components usually encode information common to all faces, such as the overall shape and structure. On the other hand, the subsequent components capture the variations that make each face unique.
By selecting the appropriate principal components, we can achieve remarkable separation and accuracy. It’s an art of balancing between the shared features and the unique characteristics of each individual.
Unleash Your Creativity
Now, it’s your turn to dive into the world of eigenfaces. You can download the code, play around with different principal components, and witness the magic of dimensional reduction in action. Unveil the secrets hidden within the data and explore new ways to improve classification accuracy.
If you’re hungry for more knowledge and want to dive deeper into the fascinating world of technology, visit Techal and satisfy your craving for tech insights.
So there you have it! The alluring world of eigenfaces and image classification awaits. Step into this captivating realm and unlock the potential of dimensionality reduction for yourself. Happy exploring!