Welcome to another installment of our deep learning series! In this video, we will explore some of the limitations of deep learning and discuss its future directions. While deep learning has made significant strides in solving complex problems, it is not without its challenges.
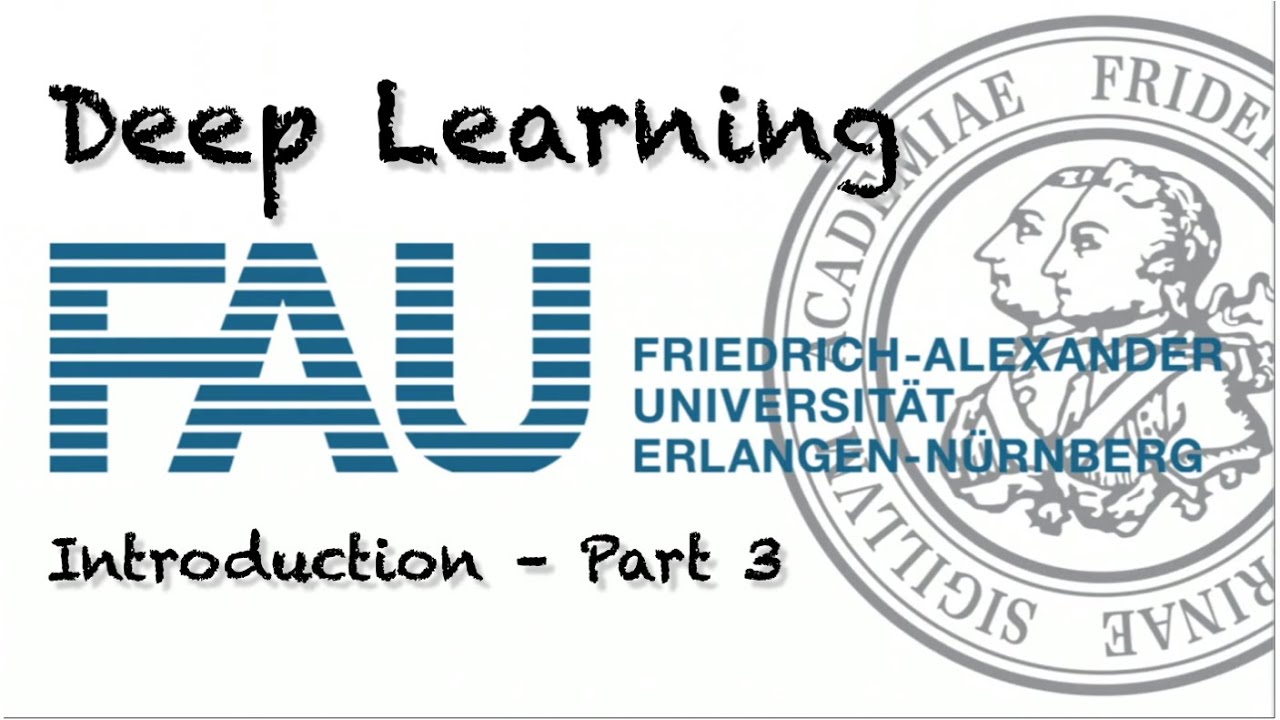
Contents
Limitations of Deep Learning
One of the main challenges in deep learning is the need for large, manually annotated datasets. These datasets are time-consuming and expensive to obtain, and they often suffer from ambiguity. Even with human annotations, errors can occur, leading to a drop in performance. Additionally, deep learning applications require massive amounts of training data, making it difficult to train models for niche or specialized tasks.
Image Captioning: Impressive but Flawed
Deep learning algorithms have yielded impressive results in tasks like image captioning. Networks can identify objects and activities with remarkable accuracy. However, they are not immune to errors. For example, while correctly identifying a baseball player or a girl in a pink dress jumping in the air, there can be errors in finer details, such as mistaking a baseball bat for an arrow or misjudging the color of an object.
Challenges with Trust and Reliability
Another challenge is the issue of trust and reliability. High-risk applications, such as autonomous vehicles, require rigorous verification and regulatory scrutiny. Deep learning algorithms, with their complex and opaque nature, make it difficult for regulators to understand how individual components work and why the system behaves a certain way. This lack of transparency can hinder the acceptance and adoption of deep learning in critical applications.
Future Directions: Learning of Algorithms
Looking ahead, one promising direction is the learning of algorithms. By expressing traditional algorithms as neural networks, we can train them for specific purposes. For example, the classical computer tomography (CT) reconstruction algorithm, which involves filtering and back projection, can be implemented as a neural network. This approach has shown promising results in improving CT image reconstruction, even in limited-angle scenarios.
By training the neural network on a small dataset, it can learn to recover lost information and reduce artifacts caused by the angular limitations of the CT scan. The beauty of this approach is that each layer of the network corresponds to a specific function in the traditional algorithm, allowing for interpretability and understanding of the underlying process.
Furthermore, combining deep learning methods with additional denoising techniques can further enhance image quality and reduce artifacts. This demonstrates the potential of deep learning to improve low contrast visualizations and overcome limitations in medical imaging applications.
FAQs
-
What are the limitations of deep learning?
Deep learning faces challenges such as the need for large annotated datasets, errors in manual annotations, and issues with trust and reliability in high-risk applications. -
Can deep learning algorithms make mistakes?
Yes, deep learning algorithms can make mistakes, especially in finer details or ambiguous scenarios. -
What is the future direction of deep learning?
The learning of algorithms is an exciting area of research, where traditional algorithms can be expressed as neural networks and trained for specific purposes.
Conclusion
While deep learning has made remarkable advancements, it is essential to understand its limitations and address them for future progress. By overcoming challenges related to data annotation, trust, and reliability, we can unlock the full potential of deep learning and pave the way for innovative applications in various fields.
Thank you for tuning in to this video! For more engaging content on the ever-evolving world of technology, visit Techal. Stay tuned for our upcoming videos, where we will delve into basic pattern recognition and machine learning. See you next time!