Welcome, my dear friends! Today, we delve into the fascinating world of mutual information. Step into the realm of discovery with me, as we uncover the intricacies of this enigmatic concept. So, fasten your seatbelts and brace yourselves for an exhilarating ride!
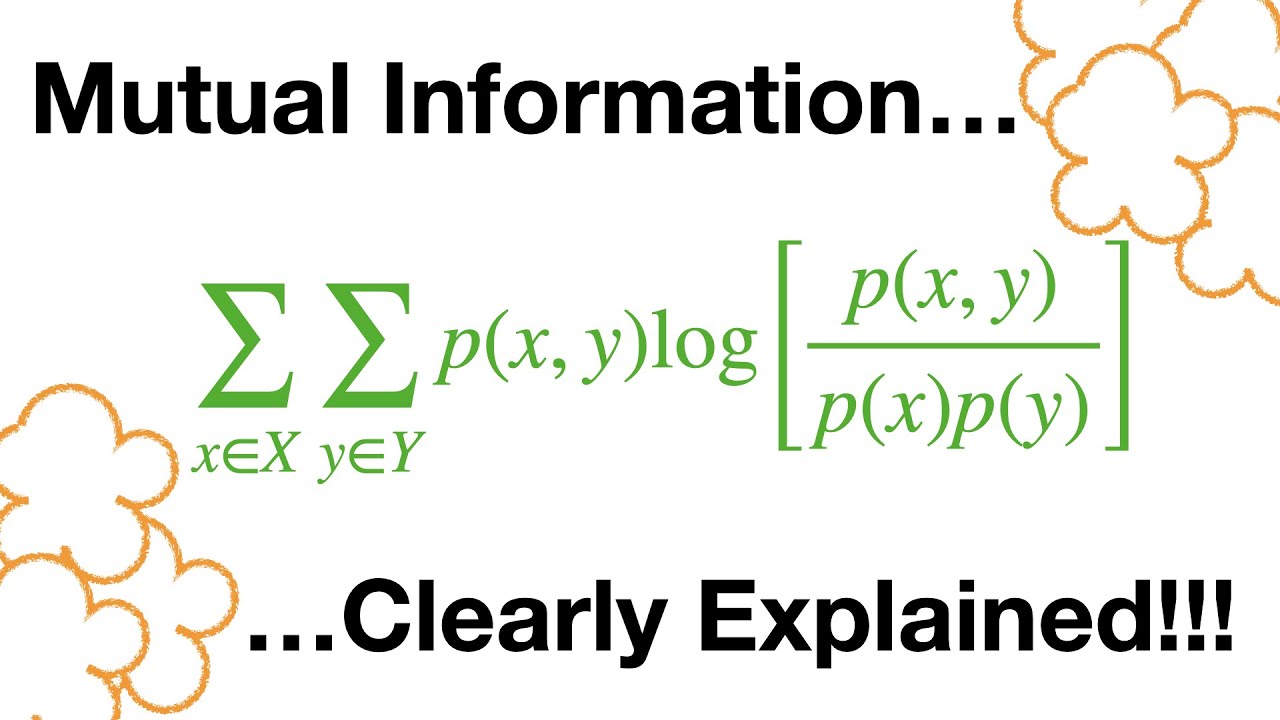
Contents
- Simplifying Complex Relationships
- Embracing the Power of Mutual Information
- Unveiling the Equation
- Deconstructing the Probabilities
- Constructing a Table of Probabilities
- Unveiling the Mutual Information
- Interpreting the Results
- Embracing Continuous Variables
- The Connection Between Mutual Information and Entropy
- Parting Words and Shameless Self-Promotion
Simplifying Complex Relationships
Imagine, if you will, a vast dataset with numerous variables, also known as features. Our goal is to simplify the data collection process by removing some variables. But how do we assess the contribution of each variable to our prediction?
Embracing the Power of Mutual Information
Introducing Mutual Information! Similar to R-squared but more versatile, Mutual Information is a numerical value that reveals the strength of the relationship between two variables. It grants us the ability to identify the variables that significantly influence our predictions.
Unveiling the Equation
Now, brace yourselves for the equation that calculates Mutual Information. Though it may appear daunting at first, fear not! We shall break it down into bite-sized pieces.
At its core, this equation showcases the power of summation. The “+” signs signify the addition of joint probabilities, which capture the likelihood of two variables occurring together. Dividing certain joint probabilities by marginal probabilities, we embark on a journey to quantify the relationship between our variables. But what are joint and marginal probabilities, you ask?
Deconstructing the Probabilities
Joint probabilities represent the likelihood of two events occurring simultaneously. Let’s take an example. Consider our dataset’s probability that someone enjoys popcorn AND loves the movie Troll 2. By dividing the count of individuals who share this preference by the total count, we obtain their joint probability.
On the other hand, marginal probabilities focus on the probability of a single event occurring. For instance, we can calculate the probability of someone not liking popcorn by dividing the count of individuals who dislike popcorn by the total count.
Constructing a Table of Probabilities
To better visualize this intricate dance between joint and marginal probabilities, we construct a table. Each column represents a variable, while each row represents an event. By populating this table with the probabilities we’ve calculated, we gain a comprehensive view of the relationships at play.
Unveiling the Mutual Information
Finally, the moment of truth arrives! With our joint and marginal probabilities in hand, we plug them into the Mutual Information equation. Uniting them with utmost precision, we witness the emergence of a numerical value that encapsulates the relationship between our variables.
Interpreting the Results
By comparing the Mutual Information values across different variables, we begin to unravel their significance. A higher Mutual Information value implies a stronger connection between the variables and can guide us in choosing the most valuable variables for our predictions.
Embracing Continuous Variables
What if we encounter a continuous variable, such as height? Fear not, for we have a solution! By converting the continuous variable into discrete categories using a histogram, we can still harness the power of Mutual Information. Each bin in the histogram becomes a category, enabling us to continue unraveling the relationships.
The Connection Between Mutual Information and Entropy
Ah, but there’s more to this tale! As observant data enthusiasts, you may notice parallels between Mutual Information and another concept called entropy. Indeed, these two share a common foundation in probability and logarithms.
Entropy focuses on surprise or change in a single variable, while Mutual Information explores the interconnectedness of changes between two variables. They dance the same dance but with slightly different rhythms, each revealing its own unique insights.
Parting Words and Shameless Self-Promotion
Before we bid adieu, my friends, I must share some exciting news! If you’re yearning for more knowledge on statistics and machine learning, Techal offers a wealth of resources. Dive into the world of PDF study guides, explore “The StatQuest Illustrated Guide to Machine Learning,” or even support us on Patreon. The possibilities are endless!
With that, we conclude this captivating journey through the depths of Mutual Information. Until we meet again, my friends, let curiosity guide your path and the quest for knowledge ignite your soul. Farewell, and may you always seek the answers that lie beyond the horizon!
Techal – Unveiling the Mysteries of Technology and Beyond.