Image Credit: Techal
Are you ready to delve into the world of statistical shape models? In this article, we will explore the fascinating realm of interventional medical image processing. This branch of technology focuses on deriving shapes from medical images and using them to enhance diagnosis and treatment. More specifically, we will discuss the intriguing concept of statistical shape models and their applications in medical image segmentation and analysis.
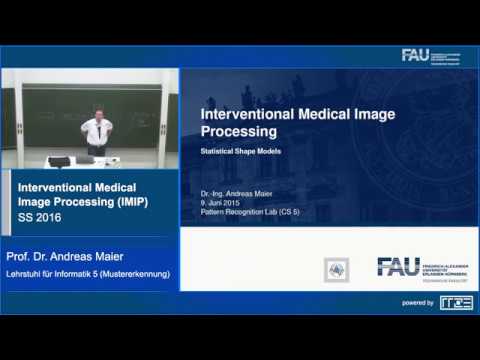
Contents
Introduction
Medical image segmentation is the process of extracting meaningful shapes from medical images. Traditional techniques often struggle with noise and artifacts, making accurate segmentation a challenge. However, statistical shape models provide a promising solution. These models capture the expected shape and variations of a specific organ or structure, allowing for a more precise and efficient segmentation process.
Statistical Shape Models – A Different Approach
Unlike other local segmentation methods, statistical shape models take a top-down approach. Instead of deriving boundaries directly from the image data, these models start with a shape model and fit it to the image. By incorporating prior knowledge of the object’s shape, statistical shape models provide a much more constrained search for the specific boundary, resulting in more accurate segmentations.
Building a Statistical Shape Model
To construct a statistical shape model, we need to follow a few essential steps. First, we define a shape representation, often using landmark points or feature descriptors. These landmarks describe specific positions on an organ or structure. Next, we establish correspondences between shapes by aligning them with respect to each other. This alignment process normalizes for rotation, translation, and scaling variations. Once we have aligned shapes, we can extract the mean shape and calculate the principal components of variation. These components represent the expected variations in shape. Finally, we can use these components and the mean shape to generate a whole continuum of different shapes.
Incorporating Appearance Models
In addition to shape information, we also need to consider the appearance of the image. Appearance models describe how an image looks at different points. This can include basic edge detection or more advanced feature extraction techniques. By integrating appearance models with shape models, we can improve the accuracy and robustness of the segmentation process.
Active Shape Models – Bridging the Gap
To fit a statistical shape model to an image, we need an optimization algorithm that combines shape and appearance models. Active shape models provide a solution. The algorithm iteratively adjusts the shape based on appearance information, updating both shape parameters and rotation, translation, and scaling parameters. This iterative process continues until convergence, resulting in a refined and accurate segmentation.
Applications of Statistical Shape Models
Statistical shape models have found numerous applications in medical image analysis. They can be used for segmenting organs like the heart, liver, and bones. In addition to segmentation, statistical shape models can also be used for shape extrapolation, allowing us to generate a moving 3D model from a limited number of 2D images. Furthermore, statistical shape models can be used to interpret shapes and perform machine learning classification based on shape features.
Conclusion
Statistical shape models are a powerful tool in the field of medical image processing. By combining shape and appearance models, these models enable accurate and efficient segmentation of organs and structures. They have been successfully applied in various medical imaging applications, facilitating diagnosis, treatment planning, and analysis. With further advancements and research, statistical shape models will continue to revolutionize the field of interventional medical image processing.
FAQs
Q: What are statistical shape models?
A: Statistical shape models capture the expected shape and variations of a specific organ or structure, enabling accurate and efficient segmentation of medical images.
Q: How do statistical shape models work?
A: Statistical shape models use prior knowledge of an object’s shape to guide the segmentation process. By starting with a shape model and fitting it to the image, these models provide a more constrained search for the specific boundary.
Q: What are the advantages of statistical shape models?
A: Statistical shape models offer more accurate segmentations compared to traditional techniques. They also enable shape extrapolation and interpretation, allowing for a deeper analysis of medical images.
Q: Can statistical shape models be used for machine learning classification?
A: Yes, statistical shape models can be used to extract shape features and perform machine learning classification, facilitating the analysis and interpretation of medical images.
Reference
- Hämäläinen, T.O., Jernström, J., 2009. Statistical shape models for 3D medical image segmentation: A review. Medical Image Analysis 13, 543–563. Read here